AI in Clinical Research
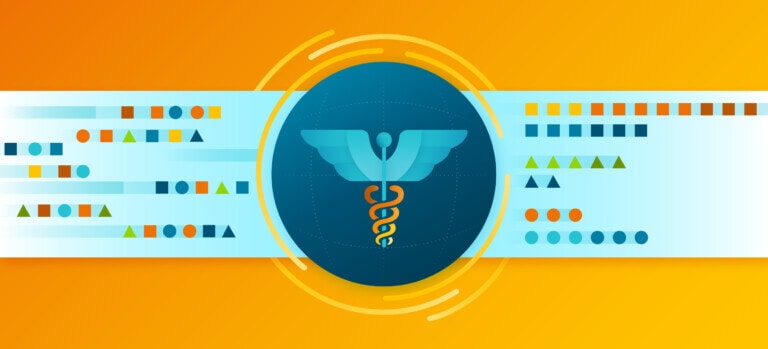
About the Advarra In Conversations With…
The future of healthcare innovation hinges on research and clinical trials. Advarra sits down with leading experts to dig into pressing issues and explore cornerstone solutions. Join us as we discuss topics and trends impacting the healthcare of tomorrow and advancing clinical research to be safer, smarter, faster.
About This Episode
In this episode, we explore the ethics and implications of artificial intelligence in clinical research.
Chapter 1: AI Crash Course
00;00;03;28 –> 00;00;23;17
Hi everyone! Welcome to the Advarra in Conversations with… podcast. Today we’re going to talk about what I hope are some interesting topics, including AI and sort of data ethics in clinical research. I’m Luke Gelinas, an IRB chair and senior director at Advarra, and I’m joined by Reid Blackman. Reid want to introduce yourself?
00;00;23;17 –> 00;00;37;17
Yeah sure, my name is Reid Blackman, I’m the CEO and founder of Virtue, an AI ethical risk consultancy. I’m also the author of Ethical Machines, a book on AI ethical risk mitigation forthcoming from Harvard Business Review press.
00;00;37;17 –> 00;00;49;00
I’m the chief ethics officer for the nonprofit government blockchain association I’m a senior advisor for Deloitte’s AI Institute, I was on a EY’s AI Advisory Board and some other things as well.
00;00;49;00 –> 00;00;54;13
Great! So, I’m excited to start our conversation, so I guess, we can just jump in get moving.
00;00;54;13 –> 00;01;05;14
in the research community we’ve been hearing sort of rumblings about AI and the ethics of AI for a long time now, this is something that’s sort of squarely within your domain expertise.
00;01;05;14 –> 00;01;20;25
I’m wondering if we could just start by having you give kind of a lay person explanation of AI maybe tailored for someone who’s never heard of it, tailored for someone like my grandma who’s you know, has no idea, very little idea about what computers do or how they work.
00;01;20;25 –> 00;01;31;26
Okay, so you know let’s start with electricity now. Okay, so um yeah so here’s a sort of crash course in what AI is putting the ethic stuff to the side obviously we’ll get to that.
00;01;31;26 –> 00;01;46;14
So, when you hear artificial intelligence or AI most people are talking about what’s called machine learning or ML, they’re basically used interchangeably by the vast majority of people, certainly in the say a business context they’re using them interchangeably.
00;01;46;14 –> 00;02;06;24
It sounds really sort of complicated and scary intellectually intimidating to a lot of people, machine learning, you know we have data scientists talking about these things are building models. But at the end of the day, the truth of the matter is that conceptually speaking it’s all relatively simple all it is software that learns by example.
00;02;06;24 –> 00;02;27;27
it’s software that learns by example so everyone is more or less familiar with software even your grandmother has interacted with software on you know at the ATM machine at the ATM. You know anything you do, on your computer it’s all software. and Okay, so it learns by example what’s an example of that? Well, let’s say you’ve got some software some photo recognition software
00;02;27;27 –> 00;02;43;22
that recognizes pictures of dogs and so you wanted to be able to upload a picture of your dog or take a picture of your dog Pepe. and your software says, “that’s Pepe” and if it’s not a picture of Pepe, it’s Pepe’s friend, then it says “not Pepe” or something like that.
00;02;43;22 –> 00;02;49;16
So how do you how do you teach the software, so to speak, what Pepe looks like? you give it a bunch of examples.
00;02;49;16 –> 00;03;05;15
fancy word for examples is data, so you just give it a bunch of photos digital photos of Pepe and the software quote unquote learns what Pepe looks like so that when you do upload or take that new picture of Pepe it says yeah that’s Pepe.
00;03;05;15 –> 00;03;15;16
And that’s the heart of it, so of course the applications can vary depending on what the examples are so if you want your software to read it, as it were, a bunch of resumes
00;03;15;16 –> 00;03;28;04
And figure out which ones are the ones that should lead to an interview then give it a bunch of examples of resumes in the past that have been judged to be worthy of an interview those that those are the examples, if you want to
00;03;28;04 –> 00;03;44;15
approve or deny people for a mortgage well give it a bunch of examples of applications that have been approved and applications that have been denied and then it will learn, hopefully, ideally, which ones are mortgage or they need which ones are not and so on and so on. So that’s all it is learning by example.
00;03;44;15 –> 00;03;56;15
Super so thinking about sort of clinical research, which is where I spend all my time and I mentioned some of our listeners will be familiar with too, what sort of applications does AI have sort of in the clinical, medical domain
00;03;56;15 –> 00;04;11;21
I’ve heard some about it being used as sort of for diagnostic purposes let’s say for figuring out who might be a good candidate for kidney liver transplant. Has it sort of reached that phase, yet where it’s doing such complex functions in the medical realm or what’s your sense there?
00;04;11;21 –> 00;04;24;20
yeah I mean I’m not going to be the best person to tell you what’s the cutting edge going on in research in medicine and health care, but I can tell you absolutely These are the kinds of applications that’s the kind of application that people are looking into so.
00;04;24;20 –> 00;04;43;22
one layer deeper about what what’s going on, when it’s learning by example, when your AI is learning by example. It’s looking at all the data that you give it, this is called trading data, and more specifically it’s looking for patterns, mathematical patterns, in all that data. So, take the Pepe example again.
00;04;43;22 –> 00;04;54;29
When you give it 1000 pictures of your dog Pepe and you say look that’s pepe it’s looking at the pixels in each picture and the mathematical relations among all those pixels.
00;04;54;29 –> 00;05;02;13
Why is that important? Well, maybe the pattern that you want to look for is not about you know, is this Pepe or not.
00;05;02;13 –> 00;05;24;26
Instead, what you’re looking for, are you uploaded or you use a bunch of data related to people who develop diabetes and people who don’t develop diabetes, and so, as it were, crawls through all that data looking for a pattern among the people who have diabetes, or who would develop diabetes and those who do not so that when you upload a new medical record
00;05;24;26 –> 00;05;35;08
It can hopefully predict with some degree of accuracy, the likelihood of that person developing diabetes in the next two years, whatever it is so.
00;05;35;08 –> 00;05;44;12
that’s more preventative it’s you know diagnosing whether someone is a likely candidate for having diabetes and the idea is supposed to be, at least in principle that.
00;05;44;12 –> 00;06;02;01
Because the because the software is learning is feeding off of is trained by all those examples, all that data It may well pick up on things that we don’t pick up on, and so it might see you know it might see that someone is at high risk of diabetes when we might not otherwise see that. that’s one thing that those researchers are trying to do with ML.
CHAPTER 2: DISCRIMINATORY AI
00;06;02;01 –> 00;06;10;00
Great fantastic I think even my grandma could understand that the basics here so thanks, maybe get into the ethics of this a little bit so.
00;06;10;00 –> 00;06;18;04
the first thing or one of the very you know common things that people worry about here is that the outputs or the conclusions or maybe the algorithms themselves.
00;06;18;04 –> 00;06;42;02
In a way, I could in some sense be discriminatory. So I want to talk about that, but I also know that you know you’re someone who, in your writing has sort of encouraged us to go beyond this concern about potentially unfair or discriminatory outputs of Ai so I wonder if you maybe you could say both a bit about you know the basic worry about discriminatory AI and then in what sense you know, we should be looking beyond it, or what other ethical concerns there are
00;06;42;02 –> 00;06;48;10
Okay, so here’s the way that I think about ethical risks and Ai. There are, there are three big risks.
00;06;48;10 –> 00;07;07;24
One is, as you mentioned discriminatory bias AI. The second one has to do with issues of black box models or unexplainable Ai. And the third one has to do with privacy violations. Those are the big three, I think, and those are the big three because the likelihood of realizing those risks is fairly high.
00;07;07;24 –> 00;07;18;28
because of the way machine learning works. because, as I like to say it’s the nature of the beast of machine learning or of the AI that we see now that those three risks are high probability.
00;07;18;28 –> 00;07;29;20
There are also loads of use case specific ethical risks. So, to give you an example outside of the healthcare industry or medicine just take self-driving cars.
00;07;29;20 –> 00;07;41;06
Self-driving cars are powered in various ways by AI, and there the ethical risks don’t have to do with discriminatory algorithms or black boxes or privacy violations, but rather with killing and maiming pedestrians.
00;07;41;06 –> 00;07;45;16
So, there’s all sorts of ways, you can use AI it’s a sort of tool right it’s a kind of tool.
00;07;45;16 –> 00;07;54;06
And so, there’s all sorts of ways, things can go wrong, depending upon what you’re trying to use it for okay so think about so there’s the three big the three big ones bias explainability and privacy.
00;07;54;06 –> 00;08;04;09
I like to say we should go beyond just talking about biased AI because there’s lots of other risks that I just articulated but there’s no doubt that it’s a phenomenally important issue, the issue of bias or discriminatory output.
00;08;04;09 –> 00;08;12;20
Quick snapshot of a medical example in healthcare. Optum was in the news, not long ago, I think this was reported in December of 2019 by the Washington Post and the Wall Street Journal.
00;08;12;20 –> 00;08;24;06
That Optum had released a model that was being used that it recommended to healthcare practitioners, doctors and nurses to pay more attention to white patients than to sicker black patients.
00;08;24;06 –> 00;08;51;12
Well, how did that happen? Well, it tried to train the AI to figure out who needs the most help, and included in that training data included in the examples, or what was relevant for making a prediction of who needs the most help were facts about who spent money on health care services in the past. The idea being that if you’re spending money on health care services, you must be needing help, but if you’re not spending money on health care services you don’t need as much help.
00;08;51;12 –> 00;08;52;00
yeah.
00;08;52;00 –> 00;08;55;26
But it turns out, for a variety of reasons, some surely discriminatory
00;08;55;26 –> 00;09;12;18
That black people spend less money on health care not because they don’t need health care, but because they just don’t have the money to spend they don’t have the insurance, and so this AI learned, so to speak, quote unquote that white people need more care than black people.
00;09;12;18 –> 00;09;16;12
Again, despite the intentions of the engineers or the data scientists.
00;09;16;12 –> 00;09;25;21
Okay, so there’s lots there’s lots of lots of things here so number one number one is well humans are bias as well, and their decision-making process and so you know we’re going to wind up with AI that’s just as biased as people
00;09;25;21 –> 00;09;27;08
Not necessarily.
00;09;27;08 –> 00;09;42;10
There are bias mitigation techniques that we can use on AI that we cannot use on people because machine learning software works differently, then people do. So, the issue is not whether we can eliminate biases: the answer to that is no, we can’t.
00;09;42;10 –> 00;09;48;06
But we can mitigate. And then the question is well using machine learning for these purposes, what’s the relevant benchmark?
00;09;48;06 –> 00;10;07;15
Let me give you another analogy so with self-driving cars. With self-driving cars, when do we think that we should just allow self-driving cars to be the rule rather than the exception? Now you might say, well, when self-driving cars are better than the average driver? Well, they’re already better than the average driver and that’s because the average driver is a really bad driver.
00;10;07;15 –> 00;10;21;28
they’re distracted, they’re Texting while driving, they’re eating while driving they’re talking about changing the song on the radio or whatever it is so. Self-driving cars are actually the bet if you think that the benchmark is average human driver, then, is outperforming that benchmark.
00;10;21;28 –> 00;10;47;18
But self-driving cars are not as good as our best drivers that it says the drivers that are not distracted, that are not eating, they’re not etc., etc., and so you might think look the relevant benchmark for safe deployment is whether it is the good driver the good human driver. Okay, why is that relevant? Well, when is it okay, to use a machine learning system that is biased, to some extent, assuming that zero biases is an impossibility?
00;10;47;18 –> 00;10;58;22
so here’s one option: when it’s less biased than the average hiring manager. Or here’s different benchmark, you might use when it’s less biased than our least biased hiring managers.
00;10;58;22 –> 00;11;13;00
We can mitigate the biases of machine learning, we can’t eliminate them and then the question is how much is enough depends upon what the appropriate benchmark is what’s the appropriate benchmark will be among other things, an ethical evaluation of what constitutes the appropriate benchmark yeah.
00;11;13;00 –> 00;11;15;13
there are loads of ways you can get discriminatory outputs.
00;11;15;13 –> 00;11;25;16
So, one example is you’ve got training data that reflects various kinds of historical discrimination, so if hiring managers weren’t hiring black people
00;11;25;16 –> 00;11;44;04
And that there’s a broadly racist explanation for that, you’re going to find that in the training data that’s the pattern is going to learn, etc., etc., OK now let’s Take, for instance, though a mortgage lending AI and it’s trained on historical data, etc., etc., and it’s going to distribute mortgages, you know let’s say it.
00;11;44;04 –> 00;11;54;02
It outputs a number between zero and one so probability of defaulting so you know point one is a 10% chance that, in a default point nine is a 90% chance that they’re going to default and.
00;11;54;02 –> 00;12;01;23
There so there’s going to be all these applications that fall between zero and one and you’ve got to set what’s called the threshold.
00;12;01;23 –> 00;12;13;17
if it’s above point three, we deny if it’s below point three, we approve Where you set that threshold is going to have an impact on various subpopulations.
00;12;13;17 –> 00;12;27;16
So, it might be the case that if you adjust your threshold you’ll have you know, an ethically acceptable impact across various subpopulations, but if you put it somewhere else it’s ethically unacceptable, it is discriminatory. Not all differential impacts are discriminatory.
00;12;27;16 –> 00;12;41;26
Right. A subset of differential impacts are discriminatory and where you set your threshold will have an impact on what that differential impact looks like so it’s not that it might not be the training data that might be involved, but where you set your threshold is going to matter.
00;12;41;26 –> 00;12;51;25
To take one last example. what you’re trying to do often is every time you have a model, you have an objective function, something that you’re trying to maximize. so let’s say you’re trying to.
00;12;51;25 –> 00;13;05;12
figure out who should get the lung, you’re doing lung transplants. And reasonably you say look, you know what I want to do, I want to maximize the number of years saved I don’t want to give a lung to the 99-year-old.
00;13;05;12–> 00;13;13;26
Because they’re not getting enough use out of it I’d rather give it to the otherwise healthy 18-year-old because I’m going to save many years of light of life, all else equal.
00;13;13;26 –> 00;13;21;13
And that’s a reasonable goal trying to maximize the quantity of years that can be saved if the lung goes to this person rather than that person.
00;13;21;13 –> 00;13;29;00
But if that’s your know in in Ai speaker and data science that’s your objective function that’s what you’re trying to maximize turns out though that.
00;13;29;00 –> 00;13;37;05
black people have worse mortality rates than white people and so you’ll wind up giving more lungs to white people, then the black people that’s not. That’s not because your training data is messed up
00;13;37;05 –> 00;13;40;18
but in fact white people tend to live longer than black people.
00;13;40;18 –> 00;13;55;12
yeah, and so the discriminatory impact is the result of the objective function, you said, not the training data. This is all just to say that there’s lots of ways of getting discriminatory outputs which also suggest that there’s lots of strategies and tactics for mitigating bias.
00;13;55;12 –> 00;14;10;04
Yeah interesting so we’ve been talking about kind of that first bucket of ethical risk, I wanted to ask you briefly about I think it was a second one, the black box. Can you speak a little bit about how the black what the black box sort of risk is and why it’s a risk?
00;14;10;04 –> 00;14;31;10
let’s just go back to Pepe, your dog. I had mentioned that what it’s doing is it’s noticing patterns in the pixels of the photographs of Pepe. So, we’re talking about thousands of pixels and thousands of mathematical relations among those pixels, in other words you’re talking about a mathematical pattern that is way too complex for you and I to comprehend,
00;14;31;10 –> 00;14;37;24
the actual patterns too mathematically complex for us to comprehend, we just don’t we can’t do that in our heads it’s just unintelligible to us.
00;14;37;24 –> 00;14;45;06
When you’re talking about labeling pictures Pepe or not Pepe, not a big deal because all you really care about is whether it’s accurate or not.
00;14;45;06 –> 00;14;50;17
In other cases, though you might care a great deal about why is it giving this output?
00;14;50;17 –> 00;14;56;19
Oh, this person, it says this person is high probability of having developing diabetes or developing cancer.
00;14;56;19 –> 00;15;20;10
I mean our experts don’t think that this person has a high likelihood of developing diabetes or cancer, but the machine picked up on some pattern or other, that is seemingly predictive of people don’t diabetes, but we don’t know why it’s making that prediction. So, what do we do? One thing that you might think is it’d be really helpful if we understood why the software why the AI made this prediction.
00;15;20;10 –> 00;15;25;23
yeah, because if we could we’d better you better at assessing it. So and so other cases there’s you know you might think.
00;15;25;23 –> 00;15;38;16
if we’re gonna deny someone say insurance, health insurance we’re going to not deny someone coverage. It seems, ethically speaking, that person is owed an explanation for why they were denied health care.
00;15;38;16 –> 00;15;45;15
that that part of respect for persons and tales that at least in some cases, not every, but in some cases, people.
00;15;45;15 –> 00;15;52;05
have a right to or deserve an explanation for why they’re being treated the way that they are particularly when the treatment is harmful.
00;15;52;05 –> 00;16;01;21
But if part of the explanation for why they’re being treated that way is because the black box that’s just a metaphor, for we can’t see inside we don’t know what’s going on.
00;16;01;21 –> 00;16;13;17
Part explanation for why you are harmed is that the black box said no that’s not a particularly satisfying explanation that doesn’t seem to satisfy that requirement for respecting people.
00;16;13;17 –> 00;16;52;20
So do you think this is kind of always a problem or sort of intrinsically a problematic? I’m thinking about sort of context, maybe we can go back to when you mentioned earlier let’s say a machine learning function for predicting diabetes. Let’s say you think you have a pretty good algorithm for doing that. I think some people argue that well we shouldn’t worry too much about trying to understand it, we should just sort of test it in randomized clinical trials like we do any other intervention for diagnosing or curing things, and if it turns out that it’s reliable, to whatever you know I showed we deem to be sufficiently reliable then accept it if not, not. So, I wonder if there are times, where this is less of a concern in your eyes?
00;16;52;20 –> 00;17;03;08
So that actually is my view. You know people go on and on about decrying the business of black boxes, but in many cases we just care about accuracy how well it does
00;17;03;08 –> 00;17;23;11
one example that I like to give is: all right look what would you prefer, a doctor or explainable software that is 75% accurate at predicting the likelihood of you developing diabetes or a black box AI that against the benchmark is 99% accurate and all else equal you might think yeah I’ll take the I want the black box model.
00;17;23;11 –> 00;17;44;28
in some cases, you just care about accuracy doesn’t matter in some high-risk cases, especially those that impact someone’s health, life, you know, including whether they may die, then plausibly you might think that using the black box model requires informed consent and it’s ethically permissible, on condition that you’ve got they’re informed consent.
00;17;44;28 –> 00;17;48;07
And it’s ethically impermissible on the condition that you do not have their informed consent
Chapter 3: AI and IRB Review
00;17;48;07 –> 00;18;02;07
yeah this sort of brings us up against another topic, I think we should cover so you’ve written it’s really interesting article titled something like “if your company uses AI you should have an IRB,” Can you sort of elaborate on the basic premise of that article?
00;18;02;07 –> 00;18;20;10
there are tools for debiasing, there are tools for explainability as well, you know explaining why the AI is giving this output. There are tools for respecting people’s privacy that say anonymize the data, use techniques like what’s called differential privacy for keeping everyone anonymous but gathering insights from the data.
00;18;20;10 –> 00;18;31;07
But there’s always going to be tough ethical decisions to be made that data scientists and engineers are not well suited to make so to give you an example.
00;18;31;07 –> 00;18;38;10
you’ve used your AI to distribute goods and services across a population let’s say it’s you’re distributing.
00;18;38;10 –> 00;18;43;29
Insurance or something like that you know you’re saying yes or no to whether or not someone gets insurance that’s the case really simple, health insurance.
00;18;43;29 –> 00;18;57;19
And you want to know okay we’ve just we’ve just sort of said yes and no to these 10,000 people so we’ve distributed, if you like, healthcare across these various subpopulations. Have we done it in a fair way?
00;18;57;19 –> 00;19;15;05
And what you might do, then, as you go to the academic literature in machine learning fairness, which is burgeoning and what you find is a couple dozen plus quantitative metrics for fairness and then you take those quantitative metrics
00;19;15;05 –> 00;19;21;20
you ask is this distribution fair by the lights of these mathematical quote unquote definitions of fairness?
00;19;21;20 –> 00;19;28;13
Now here’s the crucial part and why an IRB is so important. These mathematical definitions are not compatible with each other.
00;19;28;13 –> 00;19;43;25
You cannot score well on them all on all of them at the same time, some of them are going to require you to, for instance minimize false positives others will require you to minimize false negatives, and you can do the same thing and we’re talking about again two dozen plus metrics.
00;19;43;25 –> 00;19;54;05
so there’s this really substantive and qualitative and ethical decision to make. What’s the appropriate, again from an ethical perspective at least, what’s the ethically appropriate metric for fairness that we should use here?
00;19;54;05 –> 00;20;00;13
And that, again, data scientists are not well suited. Who is suited well? Something like the members of an IRB.
00;20;00;13 –> 00;20;12;13
yeah interesting. Can you give me an example of that where you have sort of this tension between, as you said, let’s say minimizing false positives and minimizing false negatives and what that might look like in practice?
00;20;12;13 –> 00;20;21;11
yeah so I’ll give you an example from an article by Pro Public in 2016 it’s a pretty infamous case where
00;20;21;11 –> 00;20;29;05
People judges is we’re using a software called compass to determine the risk ratings of criminal defendants.
00;20;29;05 –> 00;20;39;13
More specifically, they wanted to know what’s the risk that this person will commit a crime within the next two years, so that the judge can determine whether or not they should get bail or something along those lines.
00;20;39;13 –> 00;20;52;14
So, okay well, what would be fair In this kind of risk rating system? Now what you might want to do is, if you really, if you like, tough on crime, so to speak, you want to minimize
00;20;52;14 –> 00;21;01;140
False negatives. You really don’t want to let someone who’s a really high risk of committing a crime in the next two years to just go off just go out for the judges so yeah you’re low risk, and then the person goes off and kills someone.
00;21;01;14 –> 00;21;09;24
So, you might use a metric for fairness that that really prioritizes the importance of not letting potentially guilty people go.
00;21;09;24 –> 00;21;23;07
Yeah. On the other hand, you might think, no, no, the real the real worry here is not letting potentially letting potential criminals go free, the real worry is not letting innocent people go free.
00;21;23;07 –> 00;21;28;05
we want to minimize. false positives so minimizing false positives is your priority OK,
00;21;28;05 –> 00;21;36;08
I think my own view is that it’s more important to make sure that innocent people are not found guilty, that is that guilty people be found innocent.
00;21;36;08 –> 00;21;47;13
I recognize that that’s a substantive ethical decision. And it’s it requires investigation and data scientists don’t have anything like the training or the background to make some judgments.
00;21;47;13 –> 00;21;48;04
yeah got it.
00;21;48;04 –> 00;21;49;18
But they are making these judgments.
00;21;49;18 –> 00;21;56;10
So, in the last little bit maybe we can talk just briefly about the last category, which I think you said was privacy confidentiality.
00;21;56;10 –> 00;22;02;27
And I’d like to briefly kind of what do you think what the risks of privacy and confidentiality are with respect to AI
00;22;02;27 –> 00;22;13;26
But then I’d also love to just kind of maybe end on a more general conversation about where you think as a society we are with respect to tolerating privacy and confidentiality risk?
00;22;13;26 –> 00;22;32;24
I’m sort of struck on a daily basis by the fact that everyone now has a smartphone which can be used to locate you and track you basically at all times, almost everyone’s on social media and the companies who you know, are in control of these things have tremendous ability to sort of know what we’re up to and to look into our lives.
Chapter 4: Data Privacy and Ethics
00;22;32;24 –> 00;22;43;13
I wonder sort of, where we are, as a society with respect to our comfort level on those things? It doesn’t seem to bother most people. I know it’s sort of a big topic, but I’d love to get your thoughts on that in the few minutes that remain.
00;22;43;13 –> 00;22;48;15
so okay very quickly it’s the nature of machine learning it’s the nature of the beast.
00;22;48;15 –> 00;22;57;00
that it recognizes patterns in data and those patterns might be discriminatory and you have to set a threshold and objective function so that’s nature of the beast machine learning, they were getting up discrimination.
00;22;57;00 –> 00;23;05;24
it’s the nature of the beast of machine learning to recognize phenomenally complex patterns and then it’s the nature of the beast of machine learning that it requires data as its fuel.
00;23;05;24 –> 00;23;27;15
that’s also the nature of the beast that it makes certain kinds of predictions or inferences about people, based on all that data. So, number one, organizations are highly incentivized to collect as much data as they can about as many people as they can because all else equal, the more examples and the more data, you have the more accurate your AI is going to be. So that’s they’re incentivized, in other words, at least on some interpretations to violate people’s privacy number two.
00;23;27;15 –> 00;23;34;07
machine learning makes certain kinds of in inferences from the known to the unknown that’s the whole point of it.
00;23;34;07 –> 00;23;46;00
Now here’s an example. suppose I have geolocation from the smartphones that you mentioned, I have, I have data sets about where people go and when they go there and just as a separate data set, I have the names and addresses of therapists
00;23;46;00 –> 00;23;59;02
I feed it into my system, and I could make certain kinds of inferences about who sees the therapist you know if you know at 3:30 every day, Reid goes to this location on separately there’s this data that this location is the address of a therapist.
00;23;59;02 –> 00;24;12;24
Then you can make the probable inference that Reid sees a therapist every Wednesday at 3:30 or something along those lines. So, it’s not just the data that you train your machine learning on it’s also the new data, the data that you infer.
00;24;12;24 –> 00;24;15;22
then there is, how should we think about privacy generally.
00;24;15;22 –> 00;24;28;11
And one thing worth highlighting is that you’ve got the cyber security people who think about privacy, mostly in terms of security of the data, are you making sure that only people who should have access in fact do have access?
00;24;28;11 –> 00;24;49;20
there’s another way that people think about privacy, which is it’s just about anonymity, as long as we don’t know that you know this data is about you, then your privacy is sufficiently protected. That’s a rather passive conception of privacy in which individuals privacy is respected by virtue of it being the case that certain data is not tied to their identity.
00;24;49;20 –> 00;24;54;19
Explicitly there’s a more active conception of privacy, which is something like it’s an exercise it’s, a right that you exercise.
00;24;54;19 –> 00;25;10;02
So, in the context of AI ethics or data ethics, the right to privacy is often conceived of as something like a right to have control over your data so that’s not a passive state that’s much more of an active capacity than the state of not being identified yeah.
00;25;10;02 –> 00;25;18;04
Now, how comfortable are people? It’s hard it’s very hard to say obviously you know there’s not a small group of activists who are very worked up about this.
00;25;18;04 –> 00;25;35;06
There’s the average consumer or citizen who can’t be bothered. And everything in between, so I don’t know what the truth is that, and this is really where there’s lots to talk about I’m not convinced that privacy is the right concept, or the right, the right moral wrong to focus on.
00;25;35;06 –> 00;25;55;00
I’m less concerned about companies having the data and much more concerned with the kinds of wrong they can commit by virtue of having the data so limiting data access and things like that strikes me as a as an important strategy for mitigating the real ethical misconduct, which is downwind from possession of the data.
00;25;55;00 –> 00;26;13;21
yeah it’s really interesting, you can see the appeal of that because even as you were talking, I think, there are sort of I’m sure well known, problems with each of these approaches to privacy right, I think the notion of an anonymity, the research community is really grappling whether this is an outdated notion, because you have given enough sort of publicly available data or not even that much.
00;26;13;21 –> 00;26;25;29
Re identification is always on the table. And then, in terms of the whole you know, a right to control your data. I think, as you mentioned that earlier conversation, do people really want this right? or to what extent because you know it’s a lot of work to.
00;26;25;29 –> 00;26;27;26
it’s impossible it’s logistically impossible
00;26;27;26 –> 00;26;38;14
I think that we need to think about people and their data, not in terms of, we need to give them control, but we need to regulatory protections that people don’t need to be explicitly aware of otherwise forget it.
00;26;38;14 –> 00;26;50;00
Fascinating well, Reid, thanks so much for joining us on today’s episode of “Advarra in Conversations With…” I really enjoyed this conversation; it was great to talk and I hope we get a chance to do it again sometime.
00;26;50;00 –> 00;26;51;19
Yeah same likewise that was great!
00;26;51;19 –> 00;27;12;02
Alright, thanks all! That’s all for this week’s episode. Thanks everyone for joining, if you’re interested or found this compelling or rich, please check out Advarra’s social channels and Advarra.com for the next episode. Thanks all!